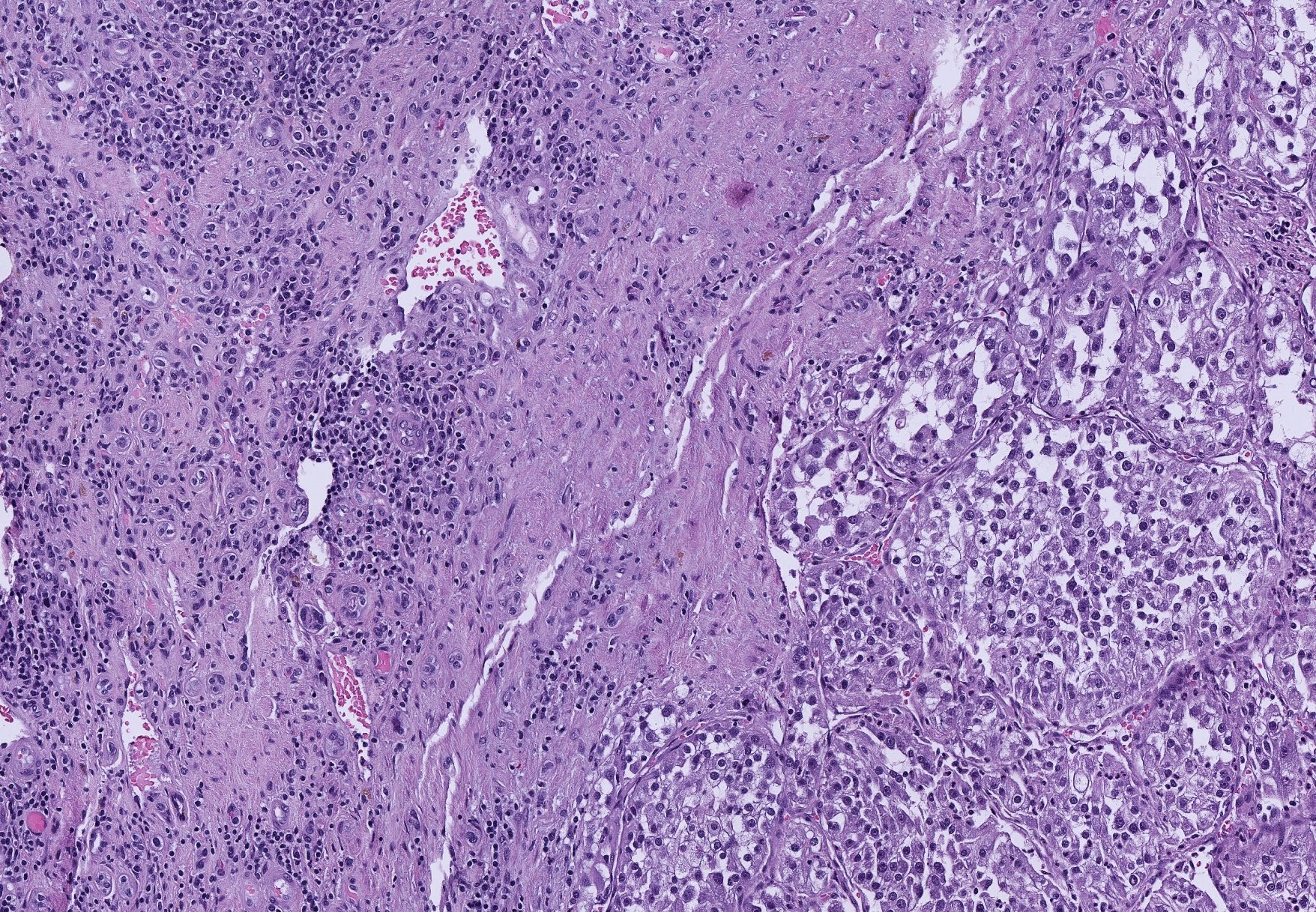
We are driven by the belief that the spatial organization of tissue provides a powerful window into cell-cell interactions, a crucial component of disease progression and response. Yet, technical challenges have left this valuable source of information untapped: human comprehension can be overwhelmed by the complexity and size of individual tissue samples while purely automated approaches are hindered by the relative scarcity of independent tissue samples. By building approaches that leverage recent advances in machine learning within the framework established by biology, we hope to establish tissue organization itself as a predictive and investigative biomarker.
Research
1. Kidney Cancer
Kidney cancer is the poster child for intra-tumor heterogeneity. By applying machine learning to tumor tissue morphology we aim to understand the molecular basis of the heterogeneity, its evolutionary implications, and to design morphogenomic biomarkers of drug response that can overcome this heterogeneity.
2. Neurodegeneration
Protein aggregation is a hallmark of neurodegeneration, yet our understanding of what processes lead to their formation remains poor. We aim to understand how the morphology and location of protein aggregates in the brain can further our understanding of these diseases, connect their classical histopathological taxonomy to the emerging one based on protein conformations and ultimately lead to better stratification of patients.
3. Radiation Oncology
The standard of care for locally advanced rectal cancer typically consists of neoadjuvant radiotherapy (RT), chemotherapy and surgery, with the latter being the primary form of treatment. Recent evidence suggests that patients that demonstrate a complete response to the neoadjuvant RT can avoid surgery permanently. This work is motivated by the hypothesis that the analysis of early post-treatment tissue morphology, and specifically the change induced by neoadjuvant RT, could lead to the discovery of biomarkers that are predictive of treatment response.
4. Deep Learning for Histopathology
We develop novel deep learning algorithms to overcome machine learning challenges unique to histopathology: massive image size, widespread heterogeneity, lack of interpretability and non-biological staining variation.
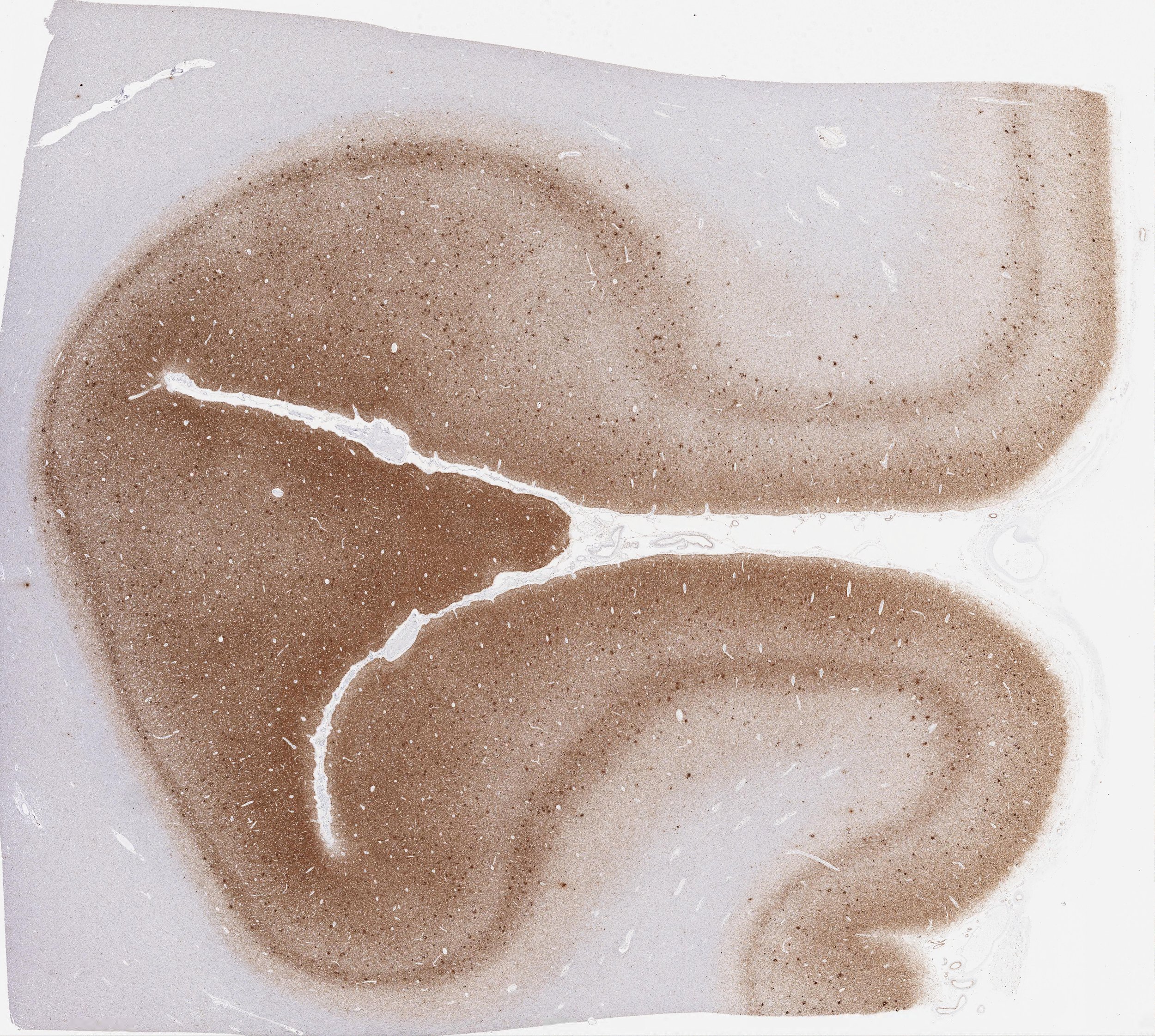
Lab Members
Satwik Rajaram
Assistant Professor
Satwik's formal training is in theoretical physics. His switch to biology occured during his Ph.D (at the University of Illinois at Urbana Champaign) as he developed statistical-physics-inspired approaches to help humans comprehend high-dimensional biological data.
Satwik's post-doctoral research in the joint labs of Drs. Altschuler and Wu (at UTSW and then at UCSF) explored the implications of cellular heterogeneity, primarily through quantitative microscopy. He developed machine learning approaches that automatically identified relevant image phenotypes - sometimes invisible to the human eye - and accurately profiled heterogeneous cellular populations. His work also explored the associated experimental design questions, such as how few cells are needed to represent a population's heterogeneity reliably.
Thuong Nguyen
Postdoctoral Researcher
Thuong completed her Ph.D degree in Mathematics at the University of Utah. Her research in the graduate program focused on developing adaptive central-upwind methods for shallow water models. She has been fascinated by computational methods, modelling, and machine learning.
As a dual affiliate with the Danuser lab, she is working on interpretable models to explain the reasoning behind the decision-making ability of machine learning models, like feature importance and accumulated local effects. The understanding of such factors contributing to likely outcomes will then help to make changes to processes.
Paul Acosta
Graduate Student
Paul received his bachelor’s degree in Biomedical Engineering at the University of Arizona. His undergraduate work focused on improving the noninvasive methods of identifying tumor acidosis by integrating chemical exchange saturation transfer (CEST) MRI and T2 exchange MRI with machine learning dimensionality reduction. Paul also engaged in work in immunology, investigating transduction events of a T cell co-receptor through a cell-free membrane reconstitution.
Currently, his graduate research focuses on investigating the relationship between tissue morphology and driver mutation functional status in clear cell renal cell carcinoma. Paul believes that there is an abundance of untapped information in tissue morphology, and through deep learning applications we can use this information to improve histopathological practices. Specifically, he is interested in being able to predict the functional status of driver mutations through tissue morphology and finding the biological features that drive this classification.
Aleksandra Nielsen
Graduate Student
Aleks received her bachelor's degree in Biotechnology, and subsequent master's degree in Bioinformatics, at Wroclaw University of Science and Technology in Poland. Her experience in computational design of small proteins allowed her to obtain the BioLAB Scholarship from the Polish-American Fulbright Commission and work abroad at UT Southwestern. Her work there led to a decision to continue graduate research at the university. Aleks' research focuses on morphology and genetics guided tumor evolution.
Sriya Veerapaneni
Graduate Student
Sriya obtained her integrated bachelor’s and master’s degree in Biological Sciences from the Indian Institute of Technology, Madras, India. Her research experiences have spanned various fields, including neuroscience and cancer biology. In her previous work, she investigated the relationships between reactive species and metabolism in colorectal cancer.
Currently, Sriya is concentrating on utilizing deep learning techniques to analyze histopathological and multiplexed images in the study of rectal cancer. Her goal is to leverage tissue morphology information to advance our understanding of rectal cancer pathology.
Jay Jasti
Data Scientist
Jay has his Ph.D in Chemical Engineering from the University of Michigan. He worked in various research organizations (Scientific Research Labs at Ford Motor Co, Unocal Science and Technology, Mobil Research and Development Corporation). More recently Jay has worked for Verizon Communications in various assignments. He recent experience has been in the general area of Fraud, Identity and Security (Identity proofing and verification, anomaly detection).
Jay is currently working on developing a kidney tumor classifier for H&E-stained Whole Slide Images using deep learning technologies.
Vipul Jarmale
Data Scientist
Vipul obtained his master's degree in Computer Science with focus on Data Science in May 2018. He is currently involved in designing and optimizing deep learning pipelines. His responsibilities also include building tools to facilitate data generation and visualization.
Hua Zhong
Computational Biologist
Hua received his master’s and Ph.D. degrees in Computer Science at New Mexico State University. His research focuses were algorithm development and applications on inference of molecular biomarkers, interactions and networks. He also worked extensively on Next-generation sequencing data downstream analysis for candidates of biological discoveries.
Hua currently works on the processing and analytical pipelines for exploring big cohorts of NGS genomic data, aiming at biological discoveries of underlying molecular mechanisms related to tumor. More specifically, he analyzes NGS data of human kidney cancer and studies relationship among genomics, morphology, clinical and pathological features.
Averi Perny
Research Assistant
Averi has a bachelor’s degree is Mathematics from University of Texas at Dallas. She joined the lab as part of the UTD Green Fellowship, working on developing better tumor grade classification models. She is currently working on improving cell classification in kidney cancer using deep learning.
Lab Alumni
Anthony Vega Postdoctoral Researcher (2019-2023) - Sr. Bio-Image Analyst (Scionics & Max Planck Institute of Molecular Cell Biology and Genetics)
Hafez Eslami Mannocheri Gradate Student (2018-2021) - Sr. Deep Learning & Computer Vision Scientist (Leica Biosystems)
Vandana Panwar Research Scientist (2018-2021) - Pathology Resident (UT Southwestern)
JOIn US!
We believe that it is a question of when, not if, machine learning revolutionizes pathology. To get there, there are several, biological, experimental and computational challenges to be overcome. We are constantly on the lookout for smart and motivated scientists (graduate students or postdocs) to join us in this effort.
- Unique multi-disciplinary training: We are a machine learning lab at one of the top academic medical centers in the world, working on problems at the cutting edge of computation and biology. Do you want to learn how to leverage your machine learning background to tackle hard problems in biology? Or maybe you want to be trained in deep learning approaches that would scale up your pathology skills? Come talk to us.
- Flexible startup-up like environment: We are a young lab in a new department committed to a fast pace. We are not afraid to break the old rules of academia to in search of what works best for our science. We've adopted open office plans with no reserved offices to maximize interactions. We have the ability to hire scientific programmers so that we can focus on algorithms+biology.
- World-class computational facilities: the BioHPC and Bioinformatics Core Facility in our department provide the strong computational and analytical infrastructure necessary to build your projects.
- High-standard of living: We offer competitive compensation that rewards industry experience and fellowships in a city with low cost of living.
For a more formal description of what we are looking for please look at our Jobs page.
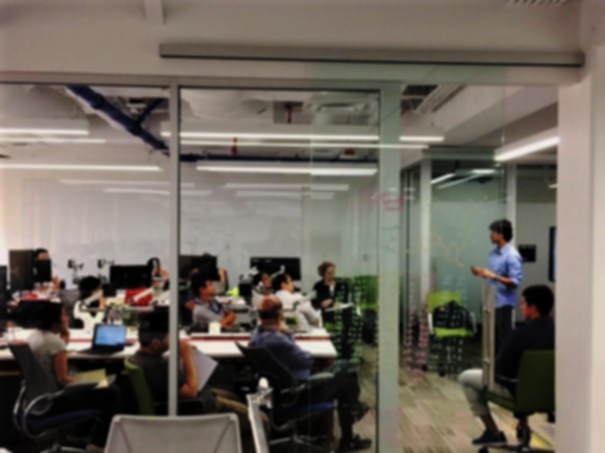